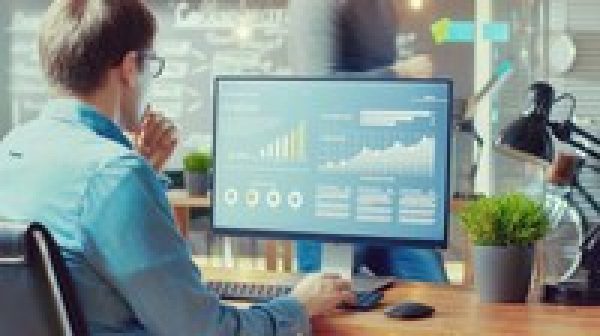
YOUR COMPLETE GUIDE TO ARTIFICIAL NEURAL NETWORKS & DEEP LEARNING IN R:
This course covers the main aspects of neural networks and deep learning. If you take this course, you can do away with taking other courses or buying books on R based data science.
In this age of big data, companies across the globe use R to sift through the avalanche of information at their disposal. By becoming proficient in neural networks and deep learning in R, you can give your company a competitive edge and boost your career to the next level!
LEARN FROM AN EXPERT DATA SCIENTIST:
My name is Minerva Singh and I am an Oxford University MPhil (Geography and Environment) graduate. I recently finished a PhD at Cambridge University.
I have +5 years of experience in analyzing real life data from different sources using data science related techniques and producing publications for international peer reviewed journals.
Over the course of my research I realized almost all the R data science courses and books out there do not account for the multidimensional nature of the topic .
This course will give you a robust grounding in the main aspects of practical neural networks and deep learning.
Instructor Details
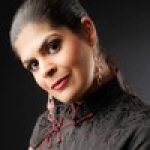
Courses : 17
Specification: Complete Deep Learning In R With Keras & Others
|
30 reviews for Complete Deep Learning In R With Keras & Others
Add a review Cancel reply
This site uses Akismet to reduce spam. Learn how your comment data is processed.
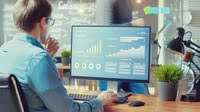
Price | $14.99 |
---|---|
Provider | |
Duration | 8 hours |
Year | 2019 |
Level | All |
Language | English |
Certificate | Yes |
Quizzes | No |

$84.99 $14.99
Paul –
Just installation instructions so far
Monica Shah –
Very detailed course. One of the very few resources on deep learning in R. It covers keras
Lisbet Ivanov –
The best and most detailed r based Ai course out there. It covers all AI packages in detail
Anit Barma –
Fully Clear Deep Learning models with Keras for Regression and Classification tasks. I am enjoying it.
Jibon Saheb –
Learning has become very interesting and easy too. it can be organized better with chapters and appropriate headings.
Reggie John –
The only detailed AI course which shows practical applications in R
Moner Protishodh –
Amazing content and examples!! The course provided a good foundation to encourage me to further my interest in Deep learning.
Nilangona –
Very good explanation of key topic areas. Logical code and examples provided to reinforce the theory covered.
Perry Carter –
This is not the most polished presentation. Lots of slip ups.
Mojar Manus –
Please keep updating as R keeps adding new packages and some older might get deprecated. Thanks for this beautiful course
Kiyer Lagi –
Thanks Dr. Minerva Singh for designing an excellent course. I have learnt a lot within a very short period
Susan Tang –
Very detailed AI course
Dennis Andersen –
succint and informative : )
Sven van Hove –
Ein gro er Strau von Methoden und Anwendungen. Praxisnah beschrieben. Etwas mehr theoretischer Hintergrund und zur Interpretation von Input und Output w re sehr sinnvoll.
Jacob Lamkey –
Very useful information although the delivery could be better
Shama –
It was good for me.
Anya Rao –
One of the few deep learning in R resources out there
Woo Ling –
Its gathering pace after a slow start. It covers the most important deep learning packages in R
Omar Andres Carmona Cortes –
The code is not explained or discussed. I believe that at least the syntax should be presented.
Anthony Smith –
Very intense course on deep learning in R. It also covers keras
Robbie Jones –
It covers a lot of R packages for deep learning
Lindsay Hiebert –
Thank you. The course is very informative and the instructor is extremely knowledgeable and moves at a very quick pace to provide explanations and examples about the workflow and concept of machine learning. It may be of value to check many of the coding examples and samples for code and data that do not seem to work well out of the box for Complete Deep Learning in R with Keras and Others . for example missing data, code, samples, CV files and packages and commands that often do not run due to packages not being installed, code not being provided, or errors being generated during the working samples. for example errors such as these > library(keras) > > data dataset mnist() Error: Installation of TensorFlow not found. > library(keras) > d2 dataset mnist() Error: Installation of TensorFlow not found. train read csv(digit train.csv) Error: ‘digit train.csv’ does not exist in current working directory > modelNnet train(default ., + training, + method net, + metric ROC, + preProcess c(‘center’, ‘scale’), + trace FALSE, + tuneLength 1, + trControl fitControl) > modelNnet Neural Network 22500 samples 23 predictor 2 classes: ‘No’, ‘Yes’ Pre processing: centered (23), scaled (23) Resampling: Cross Validated (10 fold) Summary of sample sizes: 20250, 20250, 20249, 20251, 20249, 20251, … Resampling results: ROC Sens Spec 0.7210407 0.942078 0.38115 Tuning parameter ‘size’ was held constant at a value of 1 Tuning parameter ‘decay’ was held constant at a value of 0 > > summary(modelNnet) a 23 1 1 network with 26 weights options were entropy fitting b >h1 i1 >h1 i2 >h1 i3 >h1 i4 >h1 i5 >h1 i6 >h1 i7 >h1 i8 >h1 i9 >h1 3.63 0.43 0.14 0.08 0.11 0.13 2.74 0.24 0.16 0.37 i10 >h1 i11 >h1 i12 >h1 i13 >h1 i14 >h1 i15 >h1 i16 >h1 i17 >h1 i18 >h1 i19 >h1 0.12 0.23 0.25 0.14 0.47 0.53 0.43 0.18 1.14 2.76 i20 >h1 i21 >h1 i22 >h1 i23 >h1 0.63 0.14 0.98 0.09 b >o h1 >o 0.97 2.96 > > > predNnet predict(modelNnet, testing) > cmNnet confusionMatrix(predNnet, testing$default) Error: ‘data‘ and ‘reference‘ should be factors with the same levels. > cmNnet Error: object ‘cmNnet’ not found
Anil Kumar Pandey –
Very useful and informative
Rusty –
very well explained and immensely useful
sushma Pandey –
Informative course for me
Bryan Butler –
Overall, another good course for deep learning in R. Lots of different frameworks to work with.
Jojo Statis –
Very detailed resource on AI and deep learning in R
Mahender A –
Cover all the R based AI packages
Shivam Sarin –
Not really, the tutor only explains the codes not what happens behind it. I can find these codes online. Moreover the codes are different and incomplete for some lectures. For example check section 4 ann classification with mxnet. the code uploaded is different than what is shown.
Kaushal –
Informative