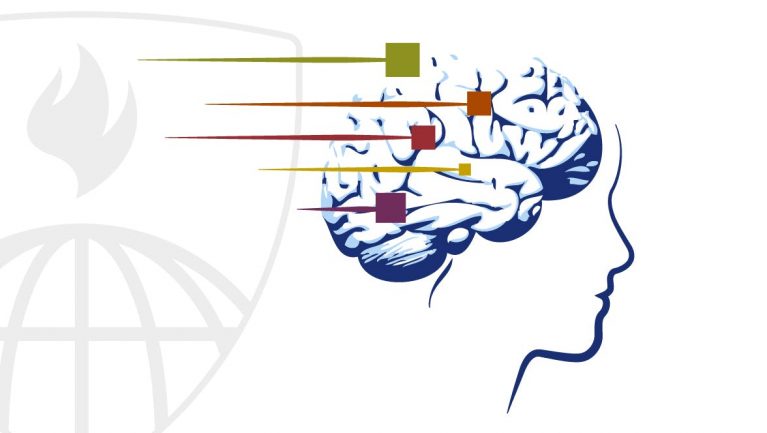
Add to wishlistAdded to wishlistRemoved from wishlist 0
Add to compare8.7/10
(Our Score)
Product is rated as #95 in category Data Science
The capstone project class will allow students to create a usable/public data product that can be used to show your skills to potential employers. Projects will be drawn from real–world problems and will be conducted with industry, government, and academic partners. The mission of The Johns Hopkins University is to educate its students and cultivate their capacity for life–long learning, to foster independent and original research, and to bring the benefits of discovery to the world.
Instructor Details
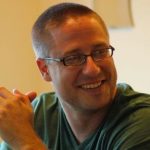
Votes: 0
Courses : 8
Specification: Data Science Capstone
|
51 reviews for Data Science Capstone
4.5 out of 5
★★★★★
★★★★★
37
★★★★★
7
★★★★★
3
★★★★★
2
★★★★★
2
Write a review
Show all
Most Helpful
Highest Rating
Lowest Rating
Add a review Cancel reply
This site uses Akismet to reduce spam. Learn how your comment data is processed.

Data Science Capstone
FREE
Praveen S –
Amazing course for Data Science Enthusiasts
Javier E S –
Excellent course
Jeremi S –
Challenging. The course could possibly offer a ‘here’s how it could be done’ ideal example after final submission and pass.
Ryan J –
Great course and fun project!
Shubham B –
Great for Beginners
Luis F P A –
Challenging, you are almost left alone by yourself, but i cannot deny that i learned a lot by doing it
Prabhakar B –
E
Samuel Q –
Great way to end the specialization because it forces students to think on their own and be resourceful. It is a totally different type of analysis than on any previous course so it was a great learning experience
Raunak S –
a very good concluding course for those have learnt the fundamentals of Data Science.
Keidzh S –
Brilliant course, the final chapter for the data science specialization. Spent lot of time making my final project, but it wirth it. Glad that I found this specialization a year ago.
Efejiro A –
Cool
Mohammad A –
This specialization was great and its capstone project was challenging and awesome.
Paul R –
The project topic itself is interesting, but longer (structured as 7 weeks); not much guidance until you find the right threads from mentors in the discussion forum from a few years ago or repeatedly google stackoverflow; it is much more technical than the rest of the course; and doesn’t really use much of what was learned during the meat of the specialization’s statistics/regression/ML courses, other than data science principles and tools (though new R libraries were needed). These issues aside, the project was an interesting challenge to complete nonetheless. Overall this specialization is now a few years old, and the plethora of 4 and 5 star reviews across all courses seem generous and out dated. Materials are not being updated, forums are a mess of years old threads with not much current activity; there is a feeling of waning interest and participation. This was clearly cutting edge material and course back in 2014 6, if JH/Coursera intend to continue offering it, the material needs some refresh and reordering, tougher grading rubrics (I saw a lot of inconsistency and poor quality which met the rubric criteria, alongside great quality work), and more active involvement from lecturers and mentors (and, please fix the typos).
Harland H –
Great NLP intro!
Remco B –
I really enjoyed this capstone project. An opportunity to dive into yet another data science area: NLP!
Wenjing L –
The final project is interesting. Text input prediction is a very flexible topic. It could be deep, or simple. I hope in the future more practical models will be introduced during the course. Now we are asked to explore it almost solely by ourselves, which usually isn’t the case at work, where one would seldom have to research on or develop something from scratch. Also I hope it will focus more on data analysis and visualization than developing an actual app. Shiny is a good tool to do interactive plotting, but not handy enough for UI development. I believe most people will never be asked to develop UI in Shiny at work. Finally I’d like to thank all the instructors who designed and delivered these 10 Data Science courses. I have learnt a lot from them.
Vibhutesh K S –
nice learning experiencd
Rodrigo P –
Hard work challenge, but great to achieve.
Nino P –
The task is really hard, but it should be. You are a data scientist now, be ready to deal with new analyses and new topics. It’s a bit tough since topic in NLP and we haven’t discussed much that in previous courses, but you will learn something new and apply the knowledge you gained in the specialization. Thank you Brian, Jeff and Roger for making this specialization.
Nikhil P –
What a journey, Gain lots of knowledge, very unique way to teach and lots of material to learn. Thank you for all your help.
Ana C E R –
It is hard, I am a grad student and took some extra time but is worth it. With this project, you learn a lot!
Chonlatit P –
Project is good for practice what you’ve learnt
Sandro R –
As other reviewers said, the Capstone is too unconnected to the rest of the specialization. In the end, there is no metric as to what makes your model successful, it’s just the Slides and the appearance of the Shiny app that counts towards the total mark. Also, the topic (Natural Language Processing) is just too unconnected to anything seen in the other courses. It was fun, but felt a bit off.
Premkumar S –
Great course, challenging enough but overall great experience and fun to learn
Samer M –
Very informative and beneficial course after going through all the previous courses.
Thej K R –
I spent 80 hrs on this course. I hated so many things. 1. There was lot of uncertainty in the course. For example we didn’t know how far to go with NLP. And I constantly came across in the forum where people were complaining about how there was 0 guidance and had no idea what to do. Saviours were those few people who put up help posts on the forum and sharded thier trecherous experience going down different paths. 3. The topic was already hard enough NLP, something I had no clue about and then there was this additional problem all the fucing time about memory. Jesus! One of the most painful courses primarily due to overload, lack of clear instructions and their refusal to edit one letter in the course since 5 years! Fuck them!
Max D –
NLP module should definitely be included into JHU Data Science specialization.
Andreas P –
Many thanks for the structured introduction to the extensive topic ‘Data Science’.
Alia E –
Like diving in without learning to swim first but man did I learn a lot.
Joshua M –
Very engaging and well designed course. Many thanks to the instructors and SwiftKey!
Neeraj A –
Feeling proud after completing all the courses under Data Science Specialization. This was not an easy task to complete especially if you are not familiar with the Statistics. Requires continuous dedication and motivation to follow and complete. Course is well designed and cover most of the topics. Its just stats part can be enhanced further to cover some basic aspects. Thanks for all the support
Glener D M –
Indispensable for those who want to master the R language along with data science. I highly recommend this 10 courses. Believe me you will not regret it.
Hathairat W –
The assignment was designed very well. I struggled and was thinking of giving up. I’m glad I didn’t. The assignment actually required all skills I learnt previously. A bit time consuming but achievable. Thank you very much!
John D M –
A capstone is typically defined as integrating key material from a course. This capstone did not require material from key courses, specifically the machine learning, regression models, and statistical inference courses. That was a great shame. Instead, it threw us into a completely new area, Natural Language Processing. There were many complaints about that, and I agree. However, it was a challenging task to explore an area in data science we didn’t touch on, and challenging in terms of the programming and enormous data file sizes. In that sense it was probably good prep for unexpected challenges in the workplace and therefore good training to make us real data scientists. Still, I would like to see the capstone rejigged to include material from the missing courses. As for NLP, some students claim it is not a useful area to study, but in my case it is exactly the right thing for me to study as I work with analyzing user queries in the form of tickets in a CRM. I found it especially trying to try to integrate some material such as Kneser Ney theory and opted for a more basic approach. My learning experience would have been better with some proper instruction in that area.
Ekaterina S –
It was a challegne for me, but also a fun! Very interesting experience in a new promising field!
Ahmed Z –
Great Course
Charbel L –
Interesting case study.
Muhammad Z H –
Thanks Professor
Benjamin M –
Very nice course and there was a lot to learn. Awesome!!!
Manuel E –
Hard, extremely satisfying.
Khalid S A –
Great Course, great experience
Carlos R S D –
I took this specialization a couple of months ago and did not comment as such. Now I turned around to remember some topics and started reading comments. I found many comments that say the final project has nothing to do with the previous 9 courses and when I did it I thought the same. Looking at it in perspective, I think the previous courses are absolutely necessary for the final project. The objective of carrying out a project with such characteristics is to apply the knowledge by oneself. The first courses of programming in R, extraction and cleaning, and exploratory analysis are fundamental to understand the problem. In this case the cleaning has to do with the transformations using regular expressions and tokenization. The exploratory analysis should be done in any data science project, otherwise you may encounter surprises when implementing the models. Statistical inference was necessary and closely linked to exploratory analysis, especially to select samples well and review distributions, since some machine learning methods may be affected by distributions. I must say that I did not see this when I took this course, but it was because of my lack of experience. Maybe there was a lack of guidance. The algorithm I used was regression on the ngrams for simplicity, time and capacity of my computer, but it could have been combined with other methods such as neural networks or svm. Implementing the model in shiny and then adjusting it because it was very heavy was also interesting. As a summary, I really liked this specialization and although it was very hard and many times I did not know how to move forward (especially in the capstone), I think the challenge was important for my learning and I was very entertained.
Elimane N –
Thank you very much for this course helpful
Jean–Philippe M –
I wish we had more training on text mining. Was not that easy! But overall the program is very interesting and challenging! Enjoyed it a lot!
Yohan A H –
Thanks for the guide but I did the hole course without instructions, there were new thing that could be tougth.
Ian C C Y –
Thrown into the deep sea and emerged alive!
Ben H –
Great finish to an excellent specialisation. It’s actually opened up some excellent career options for me and I am very grateful to the instructions and Coursera for providing the platform.
Pedro M –
Pretty cool
John K –
Poorly defined, and the course sets the student up to use the wrong tools.
Rizwan M –
its good learning. however NLP is never introduced in the course. its very hard to compute the metrics and train the model in R. Accuracy of the model is very low, as it’s not easy to train the models in R as it requires humungous data sets to get up to 95% accuracy. Neural networks, LSTM in python with tensor flow is the best choice to do the projects like this.
Rose G –
Interesting project. Not too tough if you take it slow and simple: my first version was already quite good and I earned a full score without going through the weird circumvolutions I saw other students get into. New subject I loved to learn about. It is a bit disappointing though that this does not follow the complete 10 classes: in my case, the knowledge I learnt in “Statistical inference”, “Regression Models” and “Machine Learning” was not applied in this project, whereas we could say those courses are the core of the specialization.