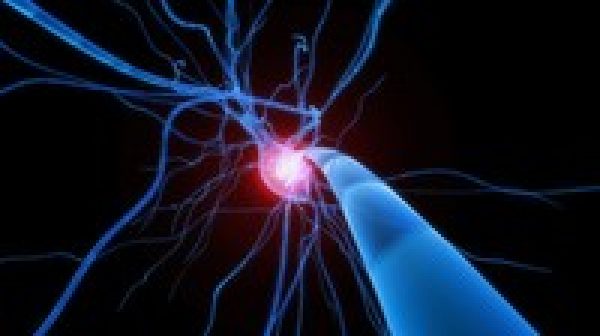
This course teaches you about one popular technique used in machine learning, data science and statistics: linear regression. We cover the theory from the ground up: derivation of the solution, and applications to real–world problems. We show you how one might code their own linear regression module in Python.
Linear regression is the simplest machine learning model you can learn, yet there is so much depth that you’ll be returning to it for years to come. That’s why it’s a great introductory course if you’re interested in taking your first steps in the fields of:
deep learning
machine learning
data science
statistics
In the first section, I will show you how to use 1–D linear regression to prove that Moore’s Law is true.
What’s that you say? Moore’s Law is not linear?
You are correct! I will show you how linear regression can still be applied.
In the next section, we will extend 1–D linear regression to any–dimensional linear regression – in other words, how to create a machine learning model that can learn from multiple inputs.
We will apply multi–dimensional linear regression to predicting a patient’s systolic blood pressure given their age and weight.
Finally, we will discuss some practical machine learning issues that you want to be mindful of when you perform data analysis, such as generalization, overfitting, train–test splits, and so on.
Instructor Details
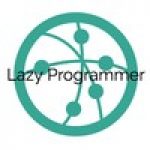
Courses : 22
Specification: Deep Learning Prerequisites: Linear Regression in Python
|
13 reviews for Deep Learning Prerequisites: Linear Regression in Python
Add a review Cancel reply
This site uses Akismet to reduce spam. Learn how your comment data is processed.

$94.99 $12.99
Dilip Upadhyay –
Good but no reference for SKLEARN package
Hannah Morgan –
I started with the NLP course and learned I should actually start here. So far, so good! Logical teacher
Ivan Radovic –
A very good introduction to linear regression. The implementation of all the concepts from the ground up in code is an excellent way to drive home the concepts. My only complaint/wish would be to have a small section addressing the assumptions of linear regression, parts of which I feel were slightly glossed over.
Kevin Huang –
This course facilitates the understanding of the math behind linear regression through actual derivations and code. The course slowly increases in complexity, helping students understand linear regression at a much higher level by the end of the course. I really enjoyed it!
Boyan –
This course is a great combination of hands on experience and in depth theoretical explanation of the mathematical concepts.
Jo o Gilberto Lamar o da Silva –
I’m really enjoying the experienced and candid feedback provided by the instructor!
Thibaut Lefebvre –
An in depth and practical course on linear regression. I highly recommend it to anyone interested in machine learning.
Felix Olowononi –
The math was well explained.
Dustin –
The information in this course is great! I wish the instructor would move a little slower on the math so it’s easier to follow, or even go step by step.
Ravikanth Parvatam –
good course
Ilija Simonovic –
This is a great course! It covers theory, math, intuition, and coding implementation of linear regression in Python. It shows how linear regression can be effectively applied even to more general problems like Moore’s law and polynomial regression. This course also explains important machine learning concepts like gradient descent, learning rates, L1, and L2 regularizations. The Instructor encourages students to ask him questions in the Q&A about any difficulty that they might have with the course material.
Pavithra K.S. –
This course really deserves 5 stars. This is the only course which explained the math behind each and every concept in the regression so far. I really enjoyed the course very much. If anybody wants to start your machine learning , this is the best course to start with. Thank you Lazy programmer
Daniel Zlatinski –
If you have most of the prerequisites, you should be able to follow along well.