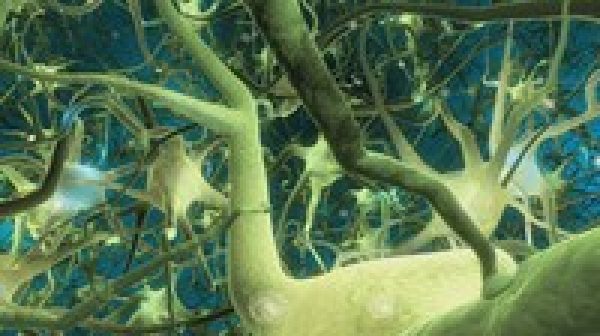
*** NOW IN TENSORFLOW 2 and PYTHON 3 ***
Learn about one of the most powerful Deep Learning architectures yet!
The Recurrent Neural Network (RNN) has been used to obtain state–of–the–art results in sequence modeling.
This includes time series analysis, forecasting and natural language processing (NLP).
Learn about why RNNs beat old–school machine learning algorithms like Hidden Markov Models.
This course will teach you:
The basics of machine learning and neurons (just a review to get you warmed up!)
Neural networks for classification and regression (just a review to get you warmed up!)
How to model sequence data
How to model time series data
How to model text data for NLP (including preprocessing steps for text)
How to build an RNN using Tensorflow 2
How to use a GRU and LSTM in Tensorflow 2
How to do time series forecasting with Tensorflow 2
How to predict stock prices and stock returns with LSTMs in Tensorflow 2 (hint: it’s not what you think!)
How to use Embeddings in Tensorflow 2 for NLP
How to build a Text Classification RNN for NLP (examples: spam detection, sentiment analysis, parts–of–speech tagging, named entity recognition)
All of the materials required for this course can be downloaded and installed for FREE. We will do most of our work in Numpy, Matplotlib, and Tensorflow. I am always available to answer your questions and help you along your data science journey.
Instructor Details
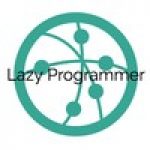
Courses : 22
Specification: Deep Learning: Recurrent Neural Networks in Python
|
5 reviews for Deep Learning: Recurrent Neural Networks in Python
Add a review Cancel reply
This site uses Akismet to reduce spam. Learn how your comment data is processed.

$94.99 $12.99
Priyanka Aishwarya –
yes
Charles Johler –
excellent!
George Herbert –
This course wasn’t necessarily what I had anticipated. It was largely what I needed.
Ivan E Sanchez Garcia –
The theoretical part of the course (GRU and LSTM) is very poor. I did a different course, so I understood it. In general is OK.
Ana Gleice da Silva Santos –
Parece que vou aprender muito