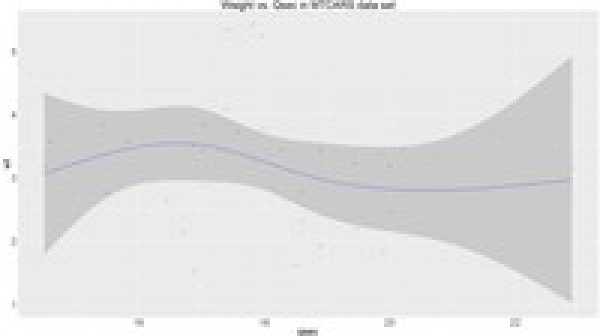
Linear Regression, GLMs and GAMs with R demonstrates how to use R to extend the basic assumptions and constraints of linear regression to specify, model, and interpret the results of generalized linear (GLMs) and generalized additive (GAMs) models. The course demonstrates the estimation of GLMs and GAMs by working through a series of practical examples from the book Generalized Additive Models: An Introduction with R by Simon N. Wood (Chapman & Hall/CRC Texts in Statistical Science, 2006). Linear statistical models have a univariate response modeled as a linear function of predictor variables and a zero mean random error term. The assumption of linearity is a critical (and limiting) characteristic. Generalized linear models (GLMs) relax this assumption of linearity. They permit the expected value of the response variable to be a smoothed (e.g. non–linear) monotonic function of the linear predictors. GLMs also relax the assumption that the response variable is normally distributed by allowing for many distributions (e.g. normal, poisson, binomial, log–linear, etc.). Generalized additive models (GAMs) are extensions of GLMs. GAMs allow for the estimation of regression coefficients that take the form of non–parametric smoothers. Nonparametric smoothers like lowess (locally weighted scatterplot smoothing) fit a smooth curve to data using localized subsets of the data. This course provides an overview of modeling GLMs and GAMs using R. GLMs, and especially GAMs, have evolved into standard statistical methodologies of considerable flexibility. The course addresses recent approaches to modeling, estimating and interpreting GAMs. The focus of the course is on modeling and interpreting GLMs and especially GAMs with R. Use of the freely available R software illustrates the practicalities of linear, generalized linear, and generalized additive models.
Instructor Details
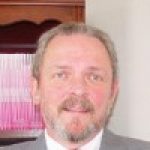
Courses : 9
Specification: Linear Regression, GLMs and GAMs with R
|
8 reviews for Linear Regression, GLMs and GAMs with R
Add a review Cancel reply
This site uses Akismet to reduce spam. Learn how your comment data is processed.

$74.99 $12.99
Kimber Phill –
I am currently enrolled in a graduate level course and the professor has skipped the foundational knowledge, assuming that we have it already. This course has been invaluable in helping me to understand the foubdations
Jim Fly –
Seems spot on to what I need to know and where I am at my knowledge level right now. Only concern is working with R since I primarily use Minitab as a statistical tool. That said, I am excited to work with R since it is a free resource.
Yonatan Aguilar –
The supplementary material is not complete and some lessons are cut or not in the right order. However, the explanations are clear and the example really help to understand the theory
koji ikeda –
very good!!
Evan John Molinelli –
This course is rich in information, but lacking in preparation. Slides were busy, the presenter was sometimes out of sync with his scripts, and concepts are used without introduction
Tanmoy Das –
An impressive course for on GLM & GAMs. I obtained in depth knowledge on them from this course.
Rosmanjawati Abdul Rahman –
So far OK..the lecture is very clear and understandable.
Juan Francisco Meraz Hernando –
So Far, so good