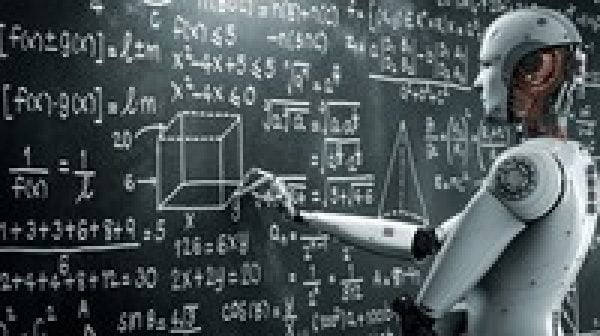
Artificial Intelligence has gained importance in the last decade with a lot depending on the development and integration of AI in our daily lives. The progress that AI has already made is astounding with the self–driving cars, medical diagnosis and even betting humans at strategy games like Go and Chess.
The future for AI is extremely promising and it isn’t far from when we have our own robotic companions. This has pushed a lot of developers to start writing codes and start developing for AI and ML programs. However, learning to write algorithms for AI and ML isn’t easy and requires extensive programming and mathematical knowledge.
Mathematics plays an important role as it builds the foundation for programming for these two streams. And in this course, we’ve covered exactly that. We designed a complete course to help you master the mathematical foundation required for writing programs and algorithms for AI and ML.
The course has been designed in collaboration with industry experts to help you breakdown the difficult mathematical concepts known to man into easier to understand concepts. The course covers three main mathematical theories: Linear Algebra, Multivariate Calculus and Probability Theory.
Linear Algebra – Linear algebra notation is used in Machine Learning to describe the parameters and structure of different machine learning algorithms. This makes linear algebra a necessity to understand how neural networks are put together and how they are operating.
Instructor Details
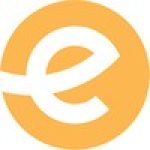
Courses : 78
Specification: Mathematical Foundation For Machine Learning and AI
|
14 reviews for Mathematical Foundation For Machine Learning and AI
Add a review Cancel reply
This site uses Akismet to reduce spam. Learn how your comment data is processed.
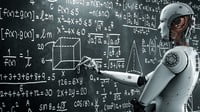
Price | $14.99 |
---|---|
Provider | |
Duration | 4.5 hours |
Year | 2018 |
Level | All |
Language | English |
Certificate | Yes |
Quizzes | No |

$49.99 $14.99
Prerna Bansal –
The quality of the introduction video is satisfactory!
Alexis Castellanos –
Great review of Linear Algebra
Jeffrey Welsh –
Good refresher!
Memi Sen –
I am expecting more example on eigen values and eigen vector.
O uz Bayram –
You need to take another foundational course to understand this course. It is a vector of formulas without any proper explanation of the background. You can’t connect these theories to machine learning, no relevant examples. Totally a big disappointment for me.
David Mills –
speed was variable. Mistakes in examples
Bangaly Camara –
Excellent
Terrance Taubes –
Very basic course.
Aniruddha Kalburgi –
spelling mistake, its indices, not indeces.
Rajesh Banka –
Matrix Multiplication and Tensors was not very clearly understood.
Dinca Andrei –
no subtitles
Niels Christian Gr nlykke –
This a great course for getting the basic tools to calculate before actually starting working on Machine Learning. Great instructor easy to understand examples
Twaine Shick –
This course is amazing over all, it sometimes doesn’t make sense, but once the instructor explains it’s helpful and makes more sense.
Shamsul Bahri –
Good for my refresher the lecture is comprehensive and the voice is crystal clear strength 5