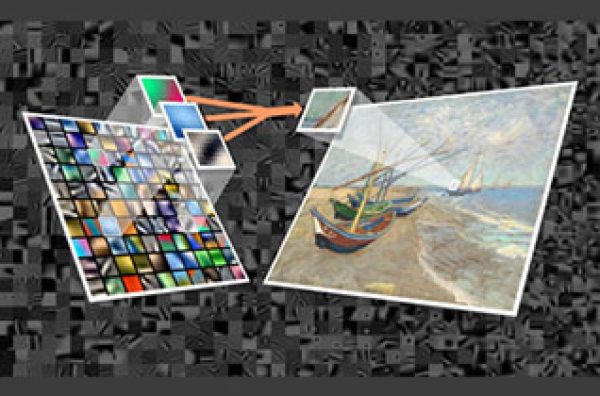
Sparse Representations in Signal and Image Processing: Fundamentals
FREE
This course introduces the fundamentals of the field of sparse representations, starting with its theoretical concepts, and systematically presenting its key achievements. We will touch on theory and numerical algorithms. Modeling data is the way we – scientists – believe that information should be explained and handled. Indeed, models play a central role in practically every task in signal and image processing. Sparse representation theory puts forward an emerging, highly effective, and universal such model. Its core idea is the description of the data as a linear combination of few building blocks – atoms – taken from a pre–defined dictionary of such fundamental elements. A series of theoretical problems arise in deploying this seemingly simple model to data sources, leading to fascinating new results in linear algebra, approximation theory, optimization, and machine learning. In this course you will learn of these achievements, which serve as the foundations for a revolution that took place in signal and image processing in recent years.
Instructor Details
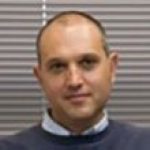
Courses : 2
Specification: Sparse Representations in Signal and Image Processing: Fundamentals
|
1 review for Sparse Representations in Signal and Image Processing: Fundamentals
Add a review Cancel reply
This site uses Akismet to reduce spam. Learn how your comment data is processed.
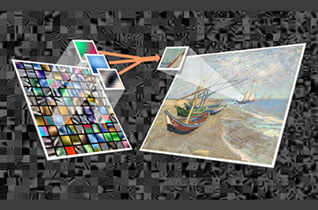
Price | Free |
---|---|
Provider | |
Duration | 27.5 hours |
Year | 2020 |
Level | Expert |
Language | English |
Certificate | Yes |
Quizzes | Yes |

FREE
Harish Ramakrishnan –
Interesting course which covers the concepts of Sparse modelling in image processing applications. Most of the course was theoretical but it did include two programming assignments based on MATLAB where we implement some of the algorithms. The requires some strong foundation in Linear algebra. Overall it is worth the time and a lot to learn.