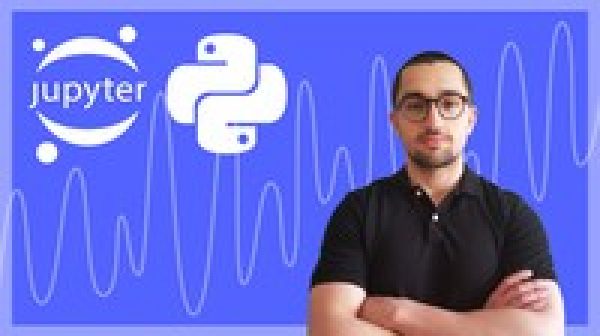
This is the only course that combines the latest statistical and deep learning techniques for time series analysis. First, the course covers the basic concepts of time series:
stationarity and augmented Dicker–Fuller test
seasonality
white noise
random walk
autoregression
moving average
ACF and PACF,
Model selection with AIC (Akaike’s Information Criterion)
Then, we move on and apply more complex statistical models for time series forecasting:
ARIMA (Autoregressive Integrated Moving Average model)
SARIMA (Seasonal Autoregressive Integrated Moving Average model)
SARIMAX (Seasonal Autoregressive Integrated Moving Average model with exogenous variables)
We also cover multiple time series forecasting with:
VAR (Vector Autoregression)
VARMA (Vector Autoregressive Moving Average model)
VARMAX (Vector Autoregressive Moving Average model with exogenous variable)
Then, we move on to the deep learning section, where we will use Tensorflow to apply different deep learning techniques for times series analysis:
Simple linear model (1 layer neural network)
DNN (Deep Neural Network)
CNN (Convolutional Neural Network)
LSTM (Long Short–Term Memory)
CNN + LSTM models
ResNet (Residual Networks)
Autoregressive LSTM
Throughout the course, you will complete more than 5 end–to–end projects in Python, with all source code available to you.
Specification: Applied Time Series Analysis in Python
|
3 reviews for Applied Time Series Analysis in Python
Add a review Cancel reply
This site uses Akismet to reduce spam. Learn how your comment data is processed.
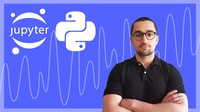
Price | $9.99 |
---|---|
Provider | |
Duration | 7 hours |
Year | 2021 |
Level | Intermediate |
Language | English ... |
Certificate | Yes |
Quizzes | No |

$84.99 $9.99
Andy Sitison –
code sections are going too fast and glazing over the stats that are a key part of the learning.
Enrique Benito Casado –
Great !! Thanks for the curse
Mingtao He –
This course is very useful. Questions can also be quickly answered by the instructor.