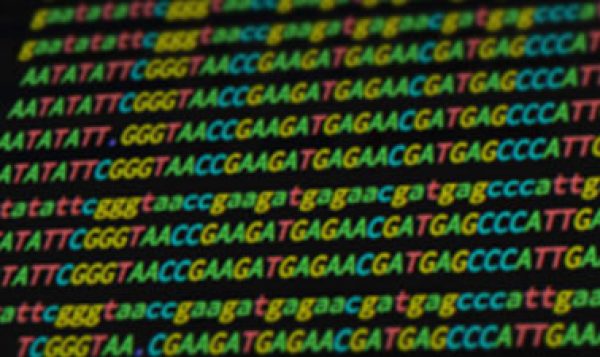
Statistical Inference and Modeling for High-throughput Experiments
FREE
In this course you’ll learn various statistics topics including multiple testing problem, error rates, error rate controlling procedures, false discovery rates, q–values and exploratory data analysis. We then introduce statistical modeling and how it is applied to high–throughput data. In particular, we will discuss parametric distributions, including binomial, exponential, and gamma, and describe maximum likelihood estimation. We provide several examples of how these concepts are applied in next generation sequencing and microarray data. Finally, we will discuss hierarchical models and empirical bayes along with some examples of how these are used in practice. We provide R programming examples in a way that will help make the connection between concepts and implementation. Given the diversity in educational background of our students we have divided the series into seven parts. You can take the entire series or individual courses that interest you. If you are a statistician you should consider skipping the first two or three courses, similarly, if you are biologists you should consider skipping some of the introductory biology lectures. Note that the statistics and programming aspects of the class ramp up in difficulty relatively quickly across the first three courses. By the third course will be teaching advanced statistical concepts such …
Instructor Details
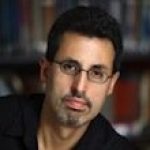
Courses : 7
Specification: Statistical Inference and Modeling for High-throughput Experiments
|
1 review for Statistical Inference and Modeling for High-throughput Experiments
Add a review Cancel reply
This site uses Akismet to reduce spam. Learn how your comment data is processed.
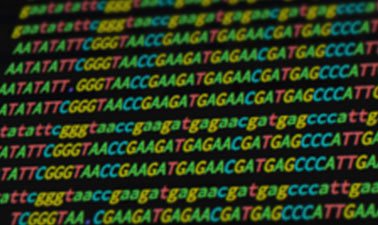
Price | Free |
---|---|
Provider | |
Duration | 12 hours |
Year | 2020 |
Level | Intermediate |
Language | English |
Certificate | Yes |
Quizzes | No |

FREE
Brandt Pence –
(Note I took these before the recent reorganization. I believe most of the material from the first few courses has remained relatively the same.)
This is the third course in the PH525 sequence offered by HarvardX. This course ended up being a bit of a surprise to me, as it was far more difficult than the previous two courses (PH525.1x and PH525.2x). Whereas previously, the lectures were at a higher level than the assignments, the assignments in this course were more difficult than the material covered in the lectures, and there was quite a bit less hand– holding compared to previous courses. Part of this may have been the material, as I had a solid background in the topics covered in the previous courses (basic statistics, R programming, and regression analyses), but I have little background in multivariate analyses.
The materials covered in this course include statistical inference for high throughput data, cluster and factor analysis, principal component analysis, hierarchical modeling, and more. I needed quite a bit of help from the discussion boards to get through some of the homework problems. Fortunately, although the EdX discussion boards are relatively poor, there was sufficient information there to get through most of the problems. I found the homework to be much less intuitive compared to previous classes, but I did learn a lot of programming and analysis tricks in this class.
Overall, four stars. This has been the most difficult course I’ve taken to this point, but getting the right answers is rewarding, and the instructors have set up the homeworks so that you have sufficient attempts to get the right answer (barely, in some cases).